The promise of the blockchain
The trust machine
The technology behind bitcoin could transform how the economy works
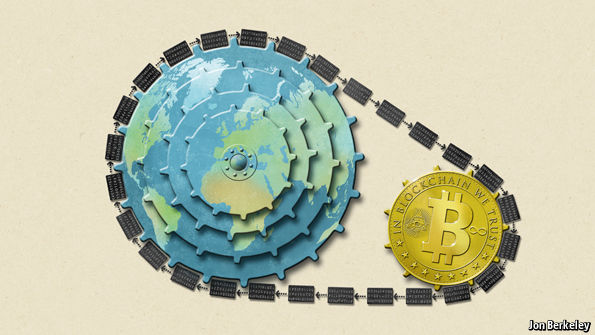
BITCOIN has a bad reputation. The decentralised digital cryptocurrency, powered by a vast computer network, is notorious for the wild fluctuations in its value, the zeal of its supporters and its degenerate uses, such as extortion, buying drugs and hiring hitmen in the online bazaars of the “dark net”.
This is unfair. The value of a bitcoin has been pretty stable, at around $250, for most of this year. Among regulators and financial institutions, scepticism has given way to enthusiasm (the European Union recently recognised it as a currency). But most unfair of all is that bitcoin’s shady image causes people to overlook the extraordinary potential of the “blockchain”, the technology that underpins it. This innovation carries a significance stretching far beyond cryptocurrency. The blockchain lets people who have no particular confidence in each other collaborate without having to go through a neutral central authority. Simply put, it is a machine for creating trust.
The blockchain food chain
To understand the power of blockchain systems, and the things they can do, it is important to distinguish between three things that are commonly muddled up, namely the bitcoin currency, the specific blockchain that underpins it and the idea of blockchains in general. A helpful analogy is with Napster, the pioneering but illegal “peer-to-peer” file-sharing service that went on line in 1999, providing free access to millions of music tracks. Napster itself was swiftly shut down, but it inspired a host of other peer-to-peer services. Many of these were also used for pirating music and films. Yet despite its dubious origins, peer-to-peer technology found legitimate uses, powering internet startups such as Skype (for telephony) and Spotify (for music streaming)—and also, as it happens, bitcoin.
The blockchain is an even more potent technology. In essence it is a shared, trusted, public ledger that everyone can inspect, but which no single user controls. The participants in a blockchain system collectively keep the ledger up to date: it can be amended only according to strict rules and by general agreement. Bitcoin’s blockchain ledger prevents double-spending and keeps track of transactions continuously. It is what makes possible a currency without a central bank.
Blockchains are also the latest example of the unexpected fruits of cryptography. Mathematical scrambling is used to boil down an original piece of information into a code, known as a hash. Any attempt to tamper with any part of the blockchain is apparent immediately—because the new hash will not match the old ones. In this way a science that keeps information secret (vital for encrypting messages and online shopping and banking) is, paradoxically, also a tool for open dealing.
Bitcoin itself may never be more than a curiosity. However blockchains have a host of other uses because they meet the need for a trustworthy record, something vital for transactions of every sort. Dozens of startups now hope to capitalise on the blockchain technology, either by doing clever things with the bitcoin blockchain or by creating new blockchains of their own (see article).
One idea, for example, is to make cheap, tamper-proof public databases—land registries, say, (Honduras and Greece are interested); or registers of the ownership of luxury goods or works of art. Documents can be notarised by embedding information about them into a public blockchain—and you will no longer need a notary to vouch for them. Financial-services firms are contemplating using blockchains as a record of who owns what instead of having a series of internal ledgers. A trusted private ledger removes the need for reconciling each transaction with a counterparty, it is fast and it minimises errors. Santander reckons that it could save banks up to $20 billion a year by 2022. Twenty-five banks have just joined a blockchain startup, called R3 CEV, to develop common standards, and NASDAQ is about to start using the technology to record trading in securities of private companies.
These new blockchains need not work in exactly the way that bitcoin’s does. Many of them could tweak its model by, for example, finding alternatives to its energy-intensive “mining” process, which pays participants newly minted bitcoins in return for providing the computing power needed to maintain the ledger. A group of vetted participants within an industry might instead agree to join a private blockchain, say, that needs less security. Blockchains can also implement business rules, such as transactions that take place only if two or more parties endorse them, or if another transaction has been completed first. As with Napster and peer-to-peer technology, a clever idea is being modified and improved. In the process, it is fast throwing off its reputation for shadiness.
New chains on the block
The spread of blockchains is bad for anyone in the “trust business”—the centralised institutions and bureaucracies, such as banks, clearing houses and government authorities that are deemed sufficiently trustworthy to handle transactions. Even as some banks and governments explore the use of this new technology, others will surely fight it. But given the decline in trust in governments and banks in recent years, a way to create more scrutiny and transparency could be no bad thing.
Drawing up regulations for blockchains at this early stage would be a mistake: the history of peer-to-peer technology suggests that it is likely to be several years before the technology’s full potential becomes clear. In the meantime regulators should stay their hands, or find ways to accommodate new approaches within existing frameworks, rather than risk stifling a fast-evolving idea with overly prescriptive rules.
The notion of shared public ledgers may not sound revolutionary or sexy. Neither did double-entry book-keeping or joint-stock companies. Yet, like them, the blockchain is an apparently mundane process that has the potential to transform how people and businesses co-operate. Bitcoin fanatics are enthralled by the libertarian ideal of a pure, digital currency beyond the reach of any central bank. The real innovation is not the digital coins themselves, but the trust machine that mints them—and which promises much more besides.